Three Principles of Effective Natural Language Query for Life Sciences
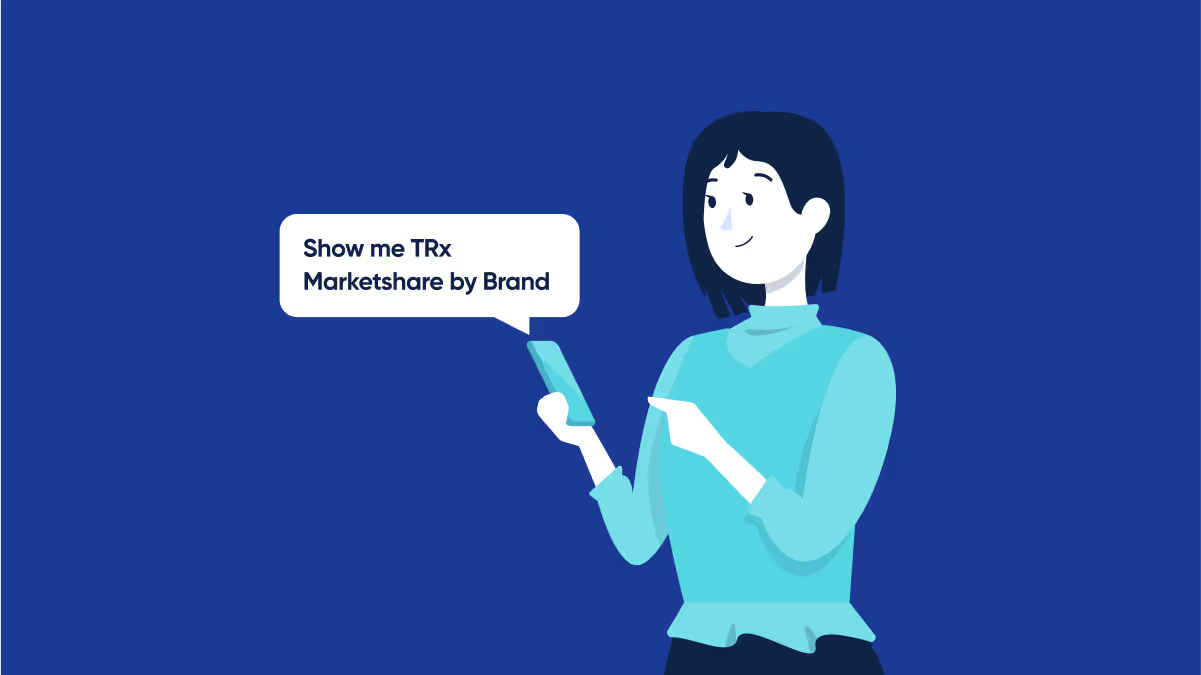
Gartner® 2022 Hype Cycle™ for Natural Language Technologies (NLT) positions natural language query (NLQ), a form of NLT, in the “Trough of Disillusionment.” All technologies go through this period after a “Peak of Inflated Expectations.” “Interest wanes as experiments and implementations fail to deliver. Investments continue only if the surviving providers improve their products to the satisfaction of early adopters.” – At that point, Gartner places the technology in the “trough” of the Hype Cycle. When “more instances of how the technology can benefit the enterprise start to crystallize and become more widely understood”*, Gartner moves the technology on the cycle to the “Slope of Enlightenment.”
WhizAI was recently recognized as a Sample Vendor in the Natural Language Query category in several Gartner Hype Cycles:
- Gartner Hype Cycle for Natural Language Technologies, 2022
- Gartner Hype Cycle for Analytics and Business Intelligence, 2022
- Gartner Hype Cycle for CRM sales Technology, 2022.
According to Gartner, NLQ is still 2 to 5 years from mainstream adoption, and one of the major drivers for NLQ, as stated by Gartner is that "adoption of NLQ continues to grow as the availability and sophistication improve, with new entrants and new features within existing platforms addressing many challenges.”Analytics platforms that leverage NLQ replaces BI dashboards with questions as the unit of work. It also replaces dependence on a data team to build dashboards and run analyses with autonomy. Although Gartner says NLQ is two to five years from mainstream adoption, WhizAI sees 100% adoption among our life sciences customers. We have perfected this with our unique domain-specific approach that understands life sciences language right from the foundation, unlike the horizontal platforms that still have a generic approach and depend on keywords and search-based queries.
But Does NLQ Work?
WhetherNLQ technology can meet those goals effectively for life sciences is a fair question – for life sciences companies – to ask. However, the answer depends on how the platform delivers NLQ. The WhizAI team has found it takes three things:
- The right NLQ engine
Different platforms approach NLQ in different ways. Some are search-based, requiring users to enter specific keywords or phrases to get accurate insights, and some platforms have attempted to bolt NLQ onto aging analytics technology.
However, a platform built from the ground up for natural language processing will provide users with a natural, conversational experience. The best approach involves leveraging machine learning, deep learning, and NLQ, as well as natural language understanding (NLU), natural language generation (NLG), and visualization to automatically provide insights most effectively. It also takes a “hybrid” approach, allowing users to fine-tune the insights the platform provides in addition to the platform continually learning and improving accuracy.
- Content, intent, and context
NLQ also has to deliver the right content, tailored to users’ native language, and understand the user so it can provide relevant, accurate, and contextual answers. For example, when a member of a life sciences sales team asks questions like, “Show me the worst sales,” the platform must understand that “sales” actually refers to “prescriptions” in this query. The platform should respond, whether the user says one word or the other, that the answer should be the same. For example, the platform needs to understand that “worst” means the user is asking for a comparison.
Platforms that are easiest to use also have capabilities such as disambiguation and slot-filling. The platform should be able to clarify, for example, if a user asks for sales in Boston if the user means the city, a territory, or a hospital. Or, if the user says, “Show me regional,” the platform will ask for more information, particularly if the user hasn’t asked a similar question in the past. These are subtleties that most NLQ solutions don’t have.
- Domain-specificity
Training the platform specifically for life sciences is also critical to how well NLQ performs. Life sciences have their own language of terms, abbreviations, acronyms, and drug and brand names. Pre training, the platform on life sciences data, readies it for real-world work in the industry.It makes implementation easier and live in weeks than with “generic” platforms that can take up to 6 months to train and test before they provide reliable results.
Where NLQ Adoption in Life SciencesReally Stands
If you evaluate NLQ solutions from all vendors, you may draw the same conclusion as Gartner that this technology is still years away from mainstream adoption.However, based on WhizAI adoption and data, NLQ provides value to thousands of life sciences users daily. One of our clients reports a 300% increase in adoption from the legacy ABI platform we replaced.
Gartner has identified challenges, suggesting that “capabilities also vary among vendors in terms of support for suggestions for the next questions to ask, spoken languages beyond English, domain and industry ontologies, ease of configuration, and how much needs to be predefined in advance versus dynamically generated.” However, WhizAI has already addressed them. For our clients, NLQ has moved beyond the Trough of Disillusionment phase of the HypeCycle, providing value to the market today.
Of course, the people using NLQ with augmented consumer platforms for life science consumers today are early adopters. Moreover, it’s providing them with quite a competitive edge by enabling data-driven decisions across all lines of business. Life sciences companies that want to remain on the cutting edge won’t wait long to join them. Contact us for more information.
*Gartner Hype Cycle Methodologies
Gartner, Hype Cycle for Natural LanguageTechnologies, 2022, Bern Elliot, Adrian Lee, 11 July 2022.
Gartner Disclaimer:
Gartner and Hype Cycle are registered trademarks and service marks of Gartner, Inc. and/or its affiliates in the U.S. and internationally and are used herein with permission. All rights reserved.Gartner does not endorse any vendor, product or service depicted in its research publications and does not advise technology users to select only those vendors with the highest ratings or other designation. Gartner research publications consist of the opinions of Gartner’s research organization and should not be construed as statements of fact. Gartner disclaims all warranties, expressed or implied, with respect to this research, including any warranties of merchantability or fitness for a particular purpose.