The Top 7 Challenges with Life Sciences Commercial Analytics
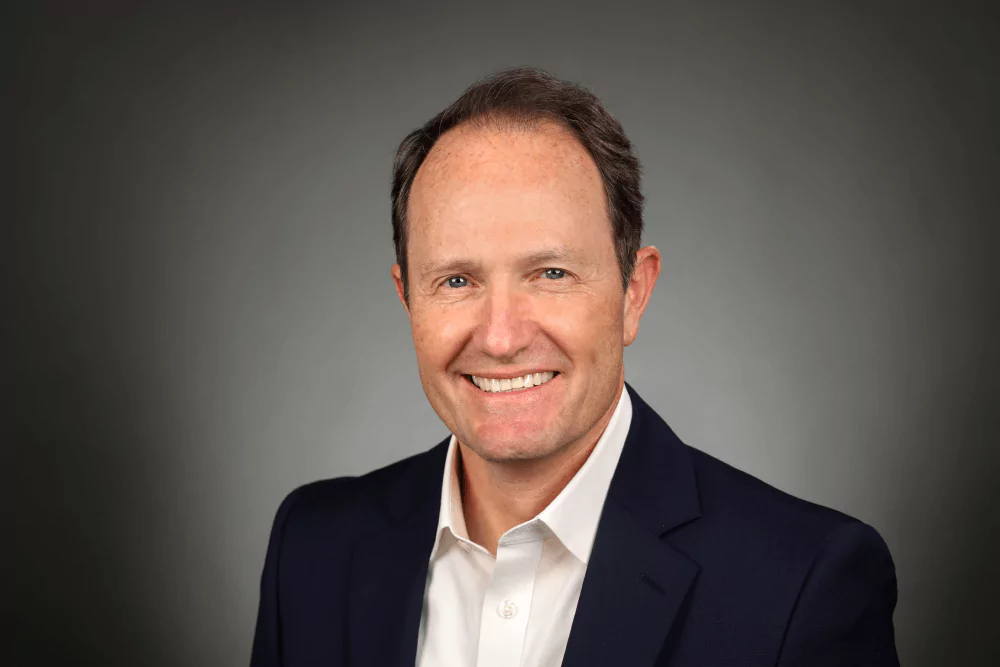
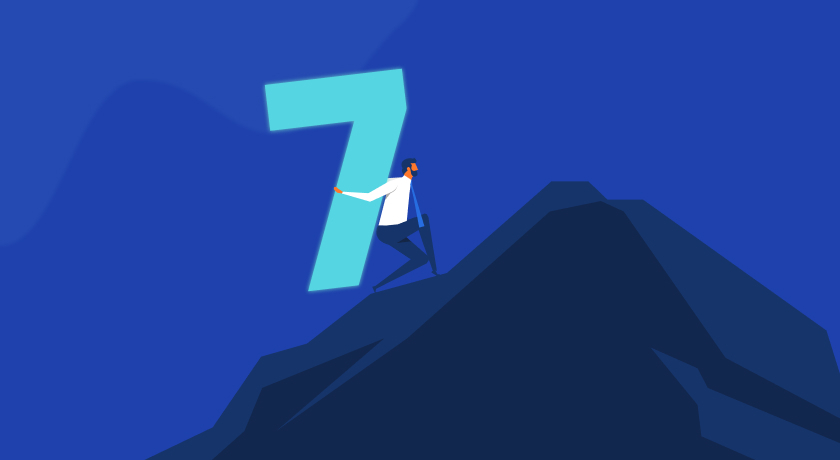
Pharmaceutical and life sciences commercial operations have a data problem. It’s not that these sales and marketing teams lack data. It’s that sales reps and marketers need a life science commercial analytics tool that allows them to access the information and insights they need, sometimes on the spot, to lead to better brand performance.
Traditionally, the standard operating procedure was for sales reps and marketers to repeat the same message over and over to everyone in their markets. However, the growing number of drugs and treatments and the rise of personalized medicine makes incorporating physician-level insights into presentations a more effective way to engage decision-makers. For example, explaining how a formulary change could specifically impact the care they provide to their patients can lead to more prescriptions and pharma company revenues.
What’s Holding Back Life Science Commercial Analytics?
While companies see the benefits of life science commercial analytics, traditional business intelligence (BI) tools haven’t been able to provide personalized insights on demand, thereby limiting commercial teams’ effectiveness.
Overcoming these seven challenges is key to an analytics tool that delivers maximum value to life science organizations.
- Time from Data to Insights
Generating actionable insights takes the right data sources, a scalable platform capable of analyzing large volumes of life sciences data, and processes that aren’t limited by the organization’s data or IT resources. Traditional BI dashboard solutions lack scalability, both in the number of data sources and the data volume they can use. Therefore, multiple dashboards may be necessary for the insights a marketing or sales team needs. They also require heavy intervention from the data team to build the dashboard, run analyses, create visualizations, and run reports. Each of these activities takes time and may result in missing out on time-sensitive opportunities.
- Domain-Specificity
Most life science commercial analytics tools that companies have deployed are simply a form of solutions developed for businesses across a range of industries. However, because life science companies have unique operations, terminology, and data types, those tools don’t deliver accurate, contextual insights or effectively show users the best next steps to take.
- Integrations
Building life science commercial analytics into legacy systems and connecting with internal and external data sources can be highly complex. In addition, some data that would make insights more valuable is siloed, and making it accessible must comply with the standards of a highly regulated industry. Integration can require a substantial investment of time and capital.
- Data Quality
The quality of data is fundamental to the quality of data insights. Errors, bias, inconsistent formatting, and other issues diminish data quality and, therefore, the reliability of the insights the tool generates.
- Required Data Expertise
Life science companies are competing for skilled data scientists from a limited talent pool. As a result, companies may operate with a smaller data or IT team than they’d prefer. Furthermore, a traditional life science commercial analytics tool requires heavy intervention from professionals with data expertise. Commercial teams typically don’t have members capable of building a dashboard or visualizations. As a result, they are forced to wait for data teams to prioritize and work through requests from all departments of their organizations. But while they wait, they may miss opportunities or overlook trends or anomalies that need their attention.
- Data Security and Governance
Making data insights available to commercial teams while protecting personal health information is a balancing act. Analytics platforms need strong data security, access control, and privacy frameworks to prevent vulnerabilities that cyberthreat actors can exploit to steal data or tamper with systems. In addition, companies must also manage data appropriately and with a high degree of transparency to build trust in the insights the platform delivers.
- User Adoption
The net result of the first six challenges has been low user adoption of life science commercial analytics tools. A long time to insights, unreliable or extraneous results, limited integrations, and heavy reliance on data and IT teams have all led to employees counting on intuition or best guesses rather than data-based decision-making. Additionally, when employees don’t use analytics tools, life science companies don’t see a return on investment from data and data analytics.
Overcoming Challenges with AI
An artificial intelligence (AI)-driven analytics platform specifically trained with life sciences data can solve the pain points that companies experience with traditional analytics tools. AI gives analytics platforms scalability and speed, reducing the time to insights to just a few seconds. Furthermore, a platform designed for life sciences teams will be enterprise-ready, with native integrations, strong security and access control, and features that support granular control for precise data governance.
Perhaps the biggest advantage AI can bring to life sciences commercial analytics is user autonomy. A tool built on a large language models (LLM) enables the AI model to understand users when they ask questions conversationally and generate accurate answers they can easily understand. Ease of use and reliable answers help build trust in the tool and increase user adoption. In fact, WhizAI experience shows that organizations can see user adoption as high as 100% since the platform is designed so any life science commercial team member can use it regardless of data science expertise.
Furthermore, when business users can access the insights they need independently, they rely on the data team less, by about 40% on average. The life science company’s data team can focus on complex analyses, and the company can reduce the total cost of data analytics ownership by as much as 50%.
The Vision for Life Sciences Commercial Analytics
Giving marketers and sales reps easy access to on-demand data analytics insights wherever they’re working can quickly enhance outcomes and help build a culture in which basing decisions on data is routine. AI-driven analytics platforms make it possible. For more information on how AI can transform life sciences commercial function, download this whitepaper from authored by Everest Group.