Causality Unleashed: Discovering Hidden Insights with Advanced Causal AI

In today’s world, for a business, making use of data to drive decisions is as important as it has ever been. At the same time, significant advancements in the field of data analysis have led to the development of many new tools for businesses. While analytical methods like regression models have long been in use, the advancement in data science, machine learning, and increased computational power has made Causal AI more accessible. In this blog, we’ll explore how Causal AI is making the difference, especially in life sciences, and how advanced causal models can provide businesses with actionable insights.
Causal vs. Correlation: Uncovering the Distinction
Correlation and Causation - While both concepts may appear similar, they have vastly different implications when it comes to decision-making.
- Correlation measures the degree to which two variables move together, but it doesn't tell us anything about the underlying cause. For instance, look at the below image pulled from Tyler Vigen’s research paper .
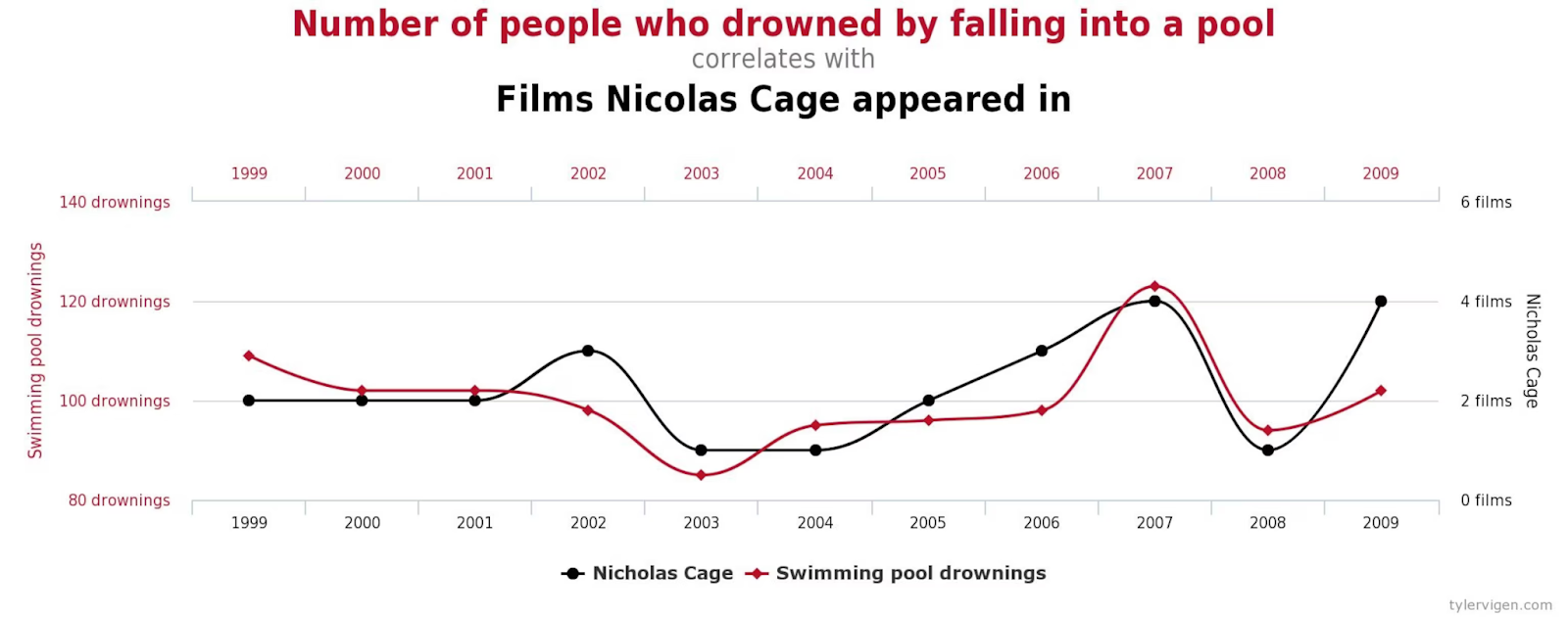
As shown in the above image, the number of people who drowned by falling into a pool correlates with the films Nicolas Cage appeared in. That does not mean the appearance of Nicolas Cage in films drives the drawing of people into a pool. Correlation does not imply causation.
- Causality, on the other hand, is about cause-and-effect relationships. Understanding true causality means identifying the factors that directly impact an outcome, allowing businesses to make actionable decisions.
Understanding this distinction is critical for businesses that rely on data to make informed decisions. Especially in the life science domain where many outcomes are highly driven by various events and activities.
Beyond Regression: Enhancing Insights with Causal AI
Traditional regression models help us identify relationships between variables—typically focusing on the association between an independent variable and a dependent outcome. However, regression has its limitations. While it can predict future trends based on existing data, it struggles to explain the causal mechanisms that influence those trends.
On the other hand, Causal AI uses advanced algorithms to model and simulate causal relationships. By incorporating domain expertise and applying techniques such as causal inference, graphical causal model, Causal AI helps reveal not just what’s happening but why it’s happening. This provides far more actionable insights, allowing businesses to simulate "what-if" scenarios and make proactive, data-backed decisions.

Real-World Applications: Transforming Decision-Making in Pharma
In industries like pharma, where innovation and speed are paramount, Causal AI can be a game-changer. Consider how pharmaceutical companies are leveraging causal modelling to improve drug development processes, optimize treatment protocols, and refine patient outcomes.
- Drug Development: By understanding the causal factors that contribute to drug efficacy and safety, pharmaceutical companies can streamline their R&D processes. Causal AI can reveal how specific molecular pathways interact with external variables like genetics, patient demographics, and environmental factors. This enables more targeted drug development, saving time and resources.
- Treatment Protocol Optimization: Causal models can also help physicians identify which treatments work best for different patient subgroups. By understanding the underlying causal relationships between patient characteristics and treatment outcomes, doctors can provide more personalized care, improving patient outcomes and reducing healthcare costs.
- Market Strategy: Causal AI allows pharma companies to better understand how market dynamics, such as competitor actions, regulatory changes, or pricing strategies, influence prescription behaviour. With this knowledge, businesses can adjust their strategies for better market positioning.
In each of these cases, Causal AI doesn’t just show correlations between variables—it helps to identify the core drivers of success and failure, enabling companies to act on insights that lead to better outcomes.
Tailored Methodologies: WhizAI's Unique Approach to Building Robust Causal Models
When it comes to Causal AI, not all approaches are created equal. WhizAI, a leader in AI-driven solutions for life sciences, brings a unique approach to building causal models that are specifically tailored for the complexities of this industry.
WhizAI’s approach is centred around domain expertise combined with cutting-edge machine learning techniques. Along with Causal analysis, it offers a host of other features like predictions, anomaly detection, key contributors etc, all bundled into the insightful ExplAIn module.
Key elements of WhizAI’s methodology include:
- Custom Data Integration: WhizAI’s models are designed to seamlessly integrate data from multiple sources, including clinical, patient-level, sales and marketing data. This holistic approach enables businesses to understand the full spectrum of causal factors at play.
- Workbench: To ensure the accuracy and trustworthiness of the outcome, WhizAI provides a workbench where data scientists and analysts can trial and error to set up the best suited template.
- Knowledge Graph: WhizAI provides an intuitive interface to capture the cause-and-effect relationship between various factors. This allows customers to build tailor made domain specific causal paths.
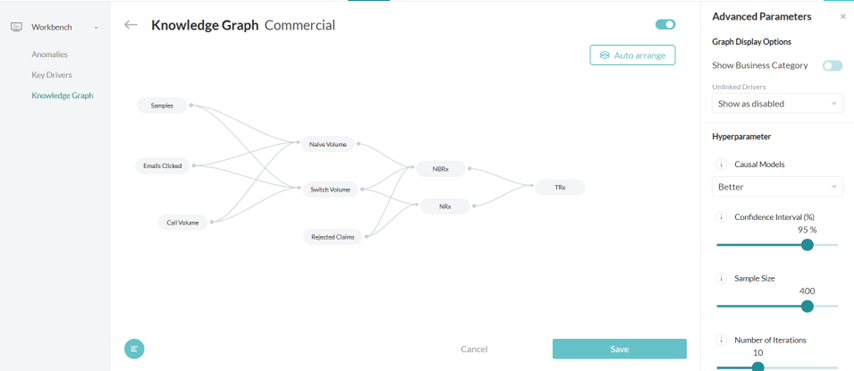
- Dynamic Causal Inference: WhizAI employs advanced dynamic causal inference techniques with options to fine tune the model. This flexibility helps in building the right model matching to the underline data and pattern.
- Lag adjustment: In many cases, the effect of a particular event takes time to show impact on the target variable. For example, physicians might start prescribing the product in a couple of weeks after the calls made by a sales person to the physician. WhizAI provisions for adjusting such lags to accurately determine the impact.
- Reach UI Illustration: One of the challenges with advanced AI models is that it is hard to comprehend and trust the results. WhizAI’s results are designed for easy interpretability, ensuring that business leaders can understand the reasoning behind the insights and make actionable decisions.
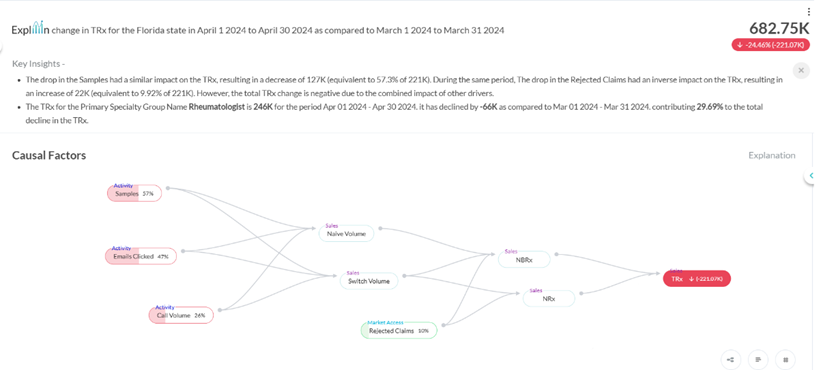

Strategic Advantage: Enabling Informed Strategies and Improved Outcomes
By leveraging the power of Causal AI, businesses—particularly in life sciences can gain a distinct strategic advantage. Causal insights allow organizations to:
- Optimize Decision-Making: Rather than simply reacting to trends, companies can make proactive, data-driven decisions that address root causes, not just symptoms.
- Improve Resource Allocation: With a clearer understanding of causal drivers, organizations can allocate resources more effectively, prioritizing initiatives that have the most significant impact.
- Enhance Innovation: By identifying the factors that lead to success in R&D, product development, or marketing, Causal AI can drive innovation in ways that traditional analytics cannot.
- Drive Growth: Whether it's increasing drug adoption rates, improving patient outcomes, or optimizing supply chains, the actionable insights from Causal AI lead to better business strategies and improved outcomes.
In conclusion, Causal AI is transforming the way businesses make decisions, providing deeper, more actionable insights that were previously out of reach. For companies in the life sciences industry, WhizAI’s innovative approach to causal modelling offers a pathway to more informed strategies, smarter resource allocation, and better overall outcomes. As the landscape becomes increasingly data-driven, understanding causality—rather than just correlation—will be a key differentiator for businesses seeking a competitive edge.
If you want to unleash the full potential of your data and gain actionable, causally informed insights, embracing ExplAIn could be your next strategic