AI-Powered Embedded Analytics is Set to Transform Life Sciences Business Intelligence
.jpg)
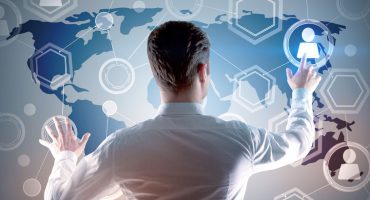
Innovation for business intelligence is speeding up. Right now, we are in the midst of a major transition. Similar to when self-service business intelligence disrupted traditional BI years ago, embedded analytics is transforming the BI landscape once again. Experts predict the embedded analytics market will reach $52 billion by 2023. That says a lot about its value to the enterprise. While embedded analytics has been around for years, this next generation of AI-powered embedded analytics is revolutionary. It’s not just embedded in workflows, it’s embedded in applications across the enterprise. It’s also contextual. All users need to do is query the system in natural language, and analytics is generated dynamically on-demand according to user needs. What makes this next evolution in BI so exciting? Let’s take a look at where embedded analytics has come from. Then, we can see how it’s a game-changer for life sciences.
What is Embedded Analytics?
Essentially, embedded analytics is the direct integration of advanced data analytics, analytic visualizations, and real-time reports into business applications. This analytic content is provided to users within their workflows. It’s also a regular part of consumer applications. To understand what it looks like, just think about when you log into your BI platform. Typically there’s an area where market trends, sales data, or other helpful charts are displayed. That’s embedded analytics. In the business environment, embedded analytics is typically seen in process applications, like CRMs, ERPs, and EHRs/EMRs. For example, in a sales application, like Veeva or Salesforce, they’re inserted in places like the account pages and tabs where users can access data like key performance indicators and sales information.
The Market is Evolving Beyond First-Gen Embedded Analytics
Embedded analytics has been around for almost a decade. When it was first introduced, the ability to access predefined reports and dashboards inside workflows was an exciting advancement. As time went on, business users were given the ability to add new, predefined reports or charts to platform pages. So now, rather than toggling between multiple applications, users were given actionable insights within their current workflows. However, as technology has advanced, this functionality has become dated. At the same time, businesses and user expectations have skyrocketed. Today’s users have all kinds of data at their fingertips thanks to smartphones. Moreover, companies increasingly expect their employees to be experts in their domains and use data to drive all of their decision-making. First-gen embedded analytics aren’t capable of meeting these high demands. Why?
- Predefined analytics and dashboards just don’t cut it anymore. The business environment is complex and fast-paced, and users need information that’s outside of narrow confines.
- Enterprises are increasingly adopting collaboration apps like MS Teams, Slack, and Zoom. As they collaborate, users want to access analytics wherever they’re working--not just in workflow apps.
Most workers switch between apps 10 times an hour, which reduces workplace productivity by 32 days every year. To help solve this issue, enter next-gen embedded analytics. This new generation enables users to access data-driven insights in the moment of every decision and every action.
Moving to the Next Level of Embedded Analytics
For a few years now, AI, machine learning, and natural language processing (NLP) have been a part of today’s business analytics, which all goes toward powering all types of decision analytics and delivering smarter, more specialized insights. Next-generation embedded analytics not only incorporates all of these technologies but also supports unanticipated use cases within a managed, seamless environment. Applications are simply embedded with self-service analytics capabilities, enabling users to ask questions about the data at the moment they occur. And the system responds with answers, even though no engineers have anticipated them. Essentially, next-gen embedded analytics is characterized by three main features:
- It’s contextual. Because it’s AI-powered, it can understand the context from a source system, as well as learn a user's preferences. For example, if a rep is viewing an HCP account in Salesforce, the embedded analytics can access and display pertinent information about writing history, market share, and proactive intelligence.
- It’s accessible from any application. There are no forms involved. There are no drag-and-drops. All it takes is embedding something like a mic or text button into an application for users to access any information from an application right where they work. For example, a user working in a home office who’s using MS Teams and speaking with a manager can easily access on-demand analytics to answer business questions during the conversation.
- Its outputs are not predefined, and they scale to user needs. AI creates visual analytics and narratives on demand to satisfy the advanced needs of business users. There aren’t any backend, manual cycles required to anticipate and define these embedded analytics. Moreover, answers are easy to understand because they are created dynamically in a way that best tells the data’s story.
Real-World Examples of Next-Gen Embedded Analytics
What can embedded analytics 2.0 do for life sciences? Let’s explore how pharmaceutical sales reps can leverage WhizAI throughout their day. On sales calls let’s say a rep is scheduled to reach out to Dr Smith and he needs the next best action. WhizAI can instantly provide the best contact method. While looking at Dr. Smith’s details in Veeva, the rep can query the system using embedded analytics, which might take the form of a search field or mic button. He’ll click the mic button and ask in natural language, “What’s the best way to contact her?” WhizAI contextually understands the rep is asking about Dr. Smith. The AI algorithm then looks at all the rep’s previous interactions with Dr Smith, knows how Dr Smith typically engages—and then the algorithm returns an answer: 77 percent of the time Dr Smith responds to emails. As the rep writes the email, he might want insights into a physician’s prescribing habits. In this case, he might ask “What is she prescribing lately?” In response, the system might generate a pie chart, which includes percentages of a competitor’s product. On the fly, because it is integrated across the enterprise, embedded analytics can be accessed from anywhere. This means that users can access it from productivity applications, like Slack. For example, if a rep is messaging a co-worker about a doctor’s prescriptions, they can simply hit a mic button and ask the system. The system’s embedded analytics will instantly deliver that data exactly where the user is working.
Provide Data-Driven Support for Every Decision
Next-gen AI-powered embedded analytics represents a revolution in business intelligence. It can answer natural language questions about any business topic, like sales or inventory, across any application. And it delivers cognitive insights based on billions of records in a matter of seconds, presenting answers in ways that employees working in unrelated departments can act on immediately. Learn more about how WhizAI can help your company make data-driven decisions and produce quantifiable results.